Customer Data and Analytics
A Data-Driven Approach to Reducing Conversion Time for Anonymous Visitors
Jul 19, 2024

Anil Bains
Founder and CEO
Introduction
Selling online has become competitive due to wide product selection, visitor data visibility, and internet penetration. Thriving in this competitive environment requires online businesses to understand their web visitors well. However, businesses have limited infrastructure to collect and process visitor data efficiently and economically.
Websites are the visitor gateways to your products and services, and a good customer experience on your website builds trust and loyalty with the brand. Traditionally, the focus had been on enhancing the customer journey of "known users" - those who have identified themselves through account creation, typically by providing their email or contact information. These loyal customers are undeniably valuable, but they represent a mere 2% of the total visitors to the website on average. A far greater untapped audience exists – the anonymous visitors. In 98% of the total visitors, anonymous visitors remain invisible to traditional marketing strategies. The key to unlocking incremental growth requires understanding and engaging with anonymous users. This article delves into how a brand can personalize and improve the website experience for anonymous visitors, who remain unknown for a large part of the funnel.
The primary challenge in engaging with anonymous visitors is understanding their preferences and tailoring the user experience to meet their needs. Let's illustrate this challenge with a scenario:
Imagine 1,000 people actively searching for products or services you offer online. Out of these potential customers, let's assume only 10% (100 people) end up on your website. Let's break this down further:
Users (2%): Companies already have some data on 2 of the 100 visitors since they are returning visitors, on average. They visit the website often, engaging with it beyond just landing on a page. They might browse product categories, view specific items, or add them to their cart.
Customer: Approximately 1 visitor from the above users makes a purchase, providing their contact information (email/phone) during the transaction. This conversion process transforms them into customers, increasing the company's customer data pool.
Anonymous Visitors (98%): The remaining 98 visitors are first-time or anonymous visitors – they have browsed the website and left without providing their email or contact information. However, they might return later, presenting a significant opportunity. To increase conversion rates, brands need a way to recognize these returning anonymous visitors and personalize their experience.

Image: Venn diagram showing visitor distribution
Average No. of Sessions to convert - a key metric to understand visitor profile and product profile
When a first-time visitor lands on your website and browses a Product Details Page (PDP) before exiting, typically, the visitor gets some information about the products and brand, etc., but the visitor isn’t totally convinced to make a purchase. These visitors might be genuinely interested but require more time for a multitude of reasons. Brands, however, need to minimize the time required for the visitor to make a purchase.
Complex, expensive, or customizable products further extend this consideration period. Premium brands with complex and customizable products typically take more sessions to convert vis-à-vis an affordable brand with standardized products. Think Capucines from Louis Vuitton bags vs. a textbook from Riley.
Price can also be a significant factor. A potential customer might be genuinely interested but find the initial price tag daunting. They might delay purchases to compare prices across brands or wait for a sale opportunity.
Ultimately, the average number of sessions after which the anonymous visitor becomes a customer varies significantly depending on the brand, product type, and target audience. A luxury watch company like Rolex will likely see a higher average than a fast-fashion retailer like Zara. Brands can understand anonymous visitor behavior by understanding the average sessions to convert and standard deviation. Here’s an example:
The number of sessions or visits required for a visitor to convert (in this case, make the purchase) can be considered a random variable following a normal distribution. Let's assume that the average number of sessions required for conversion is μ = 7 and the standard deviation is σ = 2. Approximately 68% of visitors will convert within one standard deviation (σ = 2) of the mean, between 5 and 9 sessions, 95% within two standard deviations (2σ = 4), between 3 and 11 sessions, and 99.7% between 1 and 13 sessions.
The averages and standard deviation vary across industries and brands. Understanding these customer segments becomes crucial in reducing the average sessions to convert. This statistical approach provides a quantitative understanding of the conversion journey, enabling businesses to make data-driven decisions.
To reduce the number of sessions to convert, brands can segregate their visitors into different logical buckets. For example, brands can split their visitor curve into two halves and prioritize reducing the average sessions to convert for the right half. Another common and more effective split would be a 3-part split, dividing the curve into 3 logical buckets – the bottom 16%, the middle 68%, and the top 16%.
Understanding user behavior across the curve:
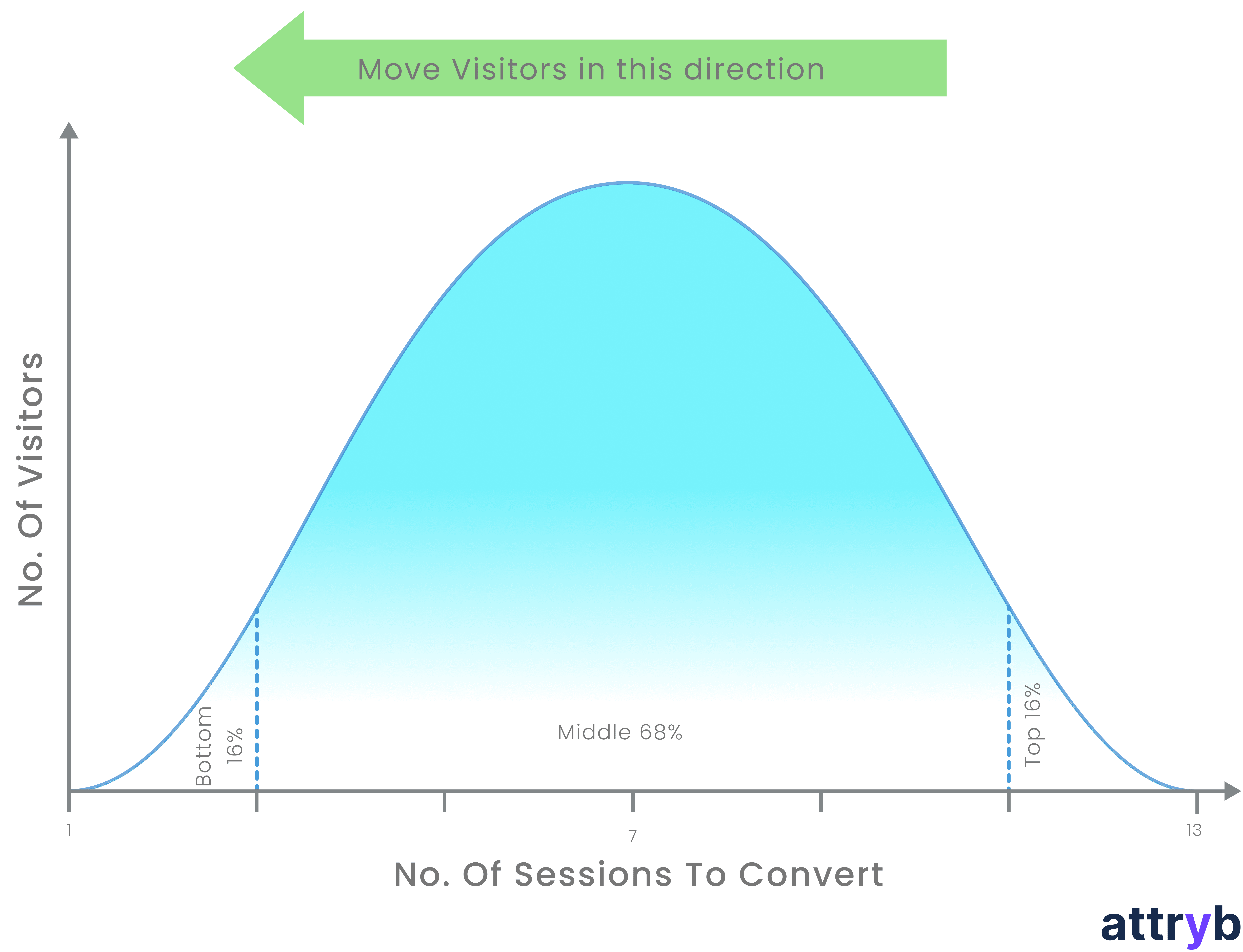
The bottom third, quick converters, are likely already acquired through existing marketing efforts. They mostly represent visitors already familiar with the brand or having a strong purchase intent. Efforts to reduce their conversion time might yield minimal gains.
The top third might have a larger pool of valuable customers who are genuinely interested but require more nurturing. They might be considering expensive products or customization options or need more convincing due to brand unfamiliarity.
By splitting the curve into three parts:
Bottom 16% of the anonymous visitors: Have a high purchase intent and convert quickly. These visitors are the brand’s best anonymous visitors with the lowest CAC. This bucket of visitors converts most likely because of one of the following reasons:
Visitors are likely familiar with the brand from offline stores or positive word-of-mouth recommendations.
They might have landed on your website after a specific product search, where the brand’s offering perfectly matches their needs.
The price point aligns with their budget, eliminating a major decision hurdle.
The middle 68% of the anonymous visitors represent visitors with high conversion potential. They're likely in the decision phase, actively considering the brand’s product but requiring more information or reassurance. Consider providing detailed product descriptions, user reviews, and buying guides that address common concerns.
Reminding them about their browsed products with personalized email campaigns or social media ads.
Targeting this segment with special promotions, discounts, or free shipping to incentivize purchases.
Implementing subtle popups displaying real-time social proof, like recent purchases or positive reviews.
Leveraging retargeting ads to reach visitors who have shown interest in your website/products but haven't converted yet.
Running limited-time offers or flash sales to create a sense of urgency and encourage visitors to convert before the opportunity disappears.
Offering loyalty programs or reward points for purchases.
Top 16% of the anonymous visitors: These have taken more than average+2*stdev sessions. This segment represents visitors who require the most effort to convert. They might be taking longer to convert since:
They might be considering high-value purchases (think luxury jewelry or complex software) and require extensive research and deliberation.
They might be facing specific roadblocks hindering their purchase decision, such as:
Price sensitivity
Unfamiliarity with the brand
Concerns regarding the exchange policy
Lack of information.
Brands can tackle these visitors by:
Tailoring efforts to address their concerns and convert them into customers.
Offering discounts or removing shipping costs for the first purchase.
Providing clear and customer-friendly exchange and return policies to alleviate worries.
Addressing product knowledge gaps with detailed descriptions, high-quality visuals, and informative FAQs.
Running targeted branding campaigns and user testimonials, which can build brand awareness and trust amongst these visitors.
While these visitors might represent potentially high-value customers due to the considered nature of their purchase, it's crucial to recognize the point of diminishing returns when investing resources. Not every visitor in this segment will convert despite targeted efforts. Some might be curious about the product or brand with no real purchase intent. Excessively pursuing these visitors can lead to wasted resources.
The key lies in identifying "reachable" high-value customers within the right segment. For example, offering extended trial periods for complex software or personalized consultations for luxury goods can address specific concerns and encourage conversion. Track conversion rates and customer lifetime value (CLTV) to determine if the effort yields a positive return on investment (ROI). Every brand and every campaign has a well-defined conversion goal, such as form-fills, purchases, downloads, etc. Any marketing efforts are in vain if:
the ratio of conversions to visitors is less than that of the middle 68% even after investing more money and effort into converting the top 16% tailing visitors or
the CLTV, which is the product of customer value and average customer lifetime, is significantly lower than that of the middle section.
For example: Imagine you invest resources into a marketing campaign targeting the top 16% of the conversion curve. Measure the conversion rate specifically for visitors exposed to the campaign (compared to a control group, if possible). A significant increase in the conversion rate indicates that the campaign is effective at bringing visitors in. Now focus on customers acquired through the campaign and calculate their CLTV. High CLTV suggests they are valuable customers with the potential to generate significant revenue over time. Compare the total cost of the campaign with the estimated revenue from the converted visitors' CLTV. If the revenue is significantly higher than the campaign cost, you have a positive ROI. Your efforts are paying off by acquiring valuable customers who bring long-term benefits. If the campaign cost outweighs the estimated revenue, you have a negative ROI. This means the interventions are not leading to conversions or high-value customers; it might be time to shift resources towards other segments.
Irrespective of how the brand decides to divide the curve and recognize the distinct pain points under each division that necessitate tailored approaches, there are universal strategies that resonate across all groups. These strategies act as hooks, drawing visitors in and nurturing them towards conversion. Three common and universal tactics are:
Personalized product recommendations: By analyzing browsing history or past purchases, brands can recommend relevant products to each visitor, such as:
For visitors in the bottom segment (16%), recommendations can introduce products frequently bought together, which they might not have considered, increasing order value. For example, if a visitor adds shoes to their cart, socks can be recommended to the visitor.
A visitor in the middle segment (68%) can be shown similar products that might pique their interest. Someone browsing athletic shoes can be shown personalized recommendations showcasing other similar shoes with different features, aiding their comparison and purchase decision.
Recommendations might address specific concerns for the topmost segment (16%). For example, if price is a barrier, suggesting similar but slightly less expensive options might nudge them towards conversion. For visitors from Tier 2 and 3 cities, typically more price-conscious, offering targeted promotions or discounts on relevant products can incentivize purchase and conversion.
Customer Experience and Brand Policies: A clear and customer-centric approach with friendly policies such as return/exchange policy fosters trust and reduces purchase apprehension across all segments. Knowing they can easily exchange an item for a different size, color, or style removes a hurdle, especially for visitors in the middle segment who might be hesitant about online purchases. For the topmost segment, a worry about receiving the wrong item or needing to exchange it can be a major roadblock. A transparent and hassle-free exchange policy addresses this concern and encourages them to convert.
Frictionless Buying Experience: Creating a seamless and user-friendly buying experience benefits all visitors. This includes a clear and intuitive website design, a streamlined checkout process, and multiple payment options. For a visitor in the bottom segment, ready to buy, a frictionless experience ensures a quick and easy checkout, reinforcing their positive perception and encouraging repeat business. For visitors in the middle segment who might be comparing options, a smooth buying experience removes frustration and keeps them engaged in the conversion process. Even for the topmost segment, where visitors might require more information or consideration, a frictionless experience allows them to navigate the website easily, find the answers they need, and ultimately convert confidently.
While not a "one-size-fits-all" solution, these strategies play a crucial role in influencing visitor behavior across the entire conversion curve. However, the type of customers under different parts of the curve will also vary significantly, which is why along with universal hooks, it is also important to tailor unique strategies to address the concerns of each segment. By engaging visitors early on, companies can potentially shorten the conversion cycle, leading to faster sales and improved customer acquisition efficiency.
Businesses need to find ways to identify and map data for these anonymous individuals while still respecting privacy and compliance regulations. Mapping this data to individual visitors can garner valuable insights into purchase intent and offline behavior.
Building Customer Profiles with Anonymous Visitor Data – Takeaways from offline retail
Imagine a shoe store scenario – a salesperson observes a customer pick up a specific shoe, check the price, and then return it to the shelf. Drawing on their experience with the brand, store location, the customer’s body language and other information they might have gathered by observing the visitor, the salesperson might recommend a different, more appropriate shoe. In essence, they’ve built a customer persona based on various data points. Similarly, online businesses can leverage data like browsing behavior, abandoned carts, devices used, and location to understand their visitors. This comprehensive online persona empowers companies to address pain points effectively, offering targeted solutions and recommendations that mirror the personalized approach of the in-store salesperson.
Available Anonymous User Data
When someone visits a website, a symphony of data collection occurs behind the scenes. This data provides valuable insights into user behavior and helps understand the audience better. This data can be categorized and broken down into four major types:
Contextual data:
Location: This could be a user's city, region, or country based on their IP address. In some cases, with user permission, it might include more precise geolocation data.
Referral Source: This identifies how a user arrived at your website (e.g., search engine, social media link, email campaign).
Time of Day: When a user visits your website can indicate their browsing habits and potential purchase intent (e.g., higher purchase rates during evenings or weekends).
Language Settings: User-preferred language settings can be used to personalize content and improve user experience.
Past Behavior: Historical data about a user's past interactions, purchases, and preferences can help businesses better understand their current context and tailor experiences accordingly
Browser Level Data:
This data provides basic information about the user's environment:
User Agent: Identifies the browser and operating system used (e.g., Chrome on Windows 10).
Screen Resolution: Provides details on the user's screen size (e.g., 1920x1080 pixels).
IP Address: Reveals the user's approximate location (city, country) based on their internet service provider. Many companies leverage a common approach: collecting Personally Identifiable Information (PII) like email addresses or phone numbers and then supplementing this data with IP address matching. In some cases, IP addresses too are considered PII. Regulations like GDPR mandate clear user consent for data collection beyond basic website functionality.
Clickstream Data:
This data tracks the user's journey through your website:
Page Views: Records which pages the user visits and for how long they stay on each page.
Links Clicked: Identifies the specific links the user interacts with on your website.
Form Interactions: Captures information submitted through forms, such as search queries or contact details (if provided).
Scroll Depth: Tracks how far down a page the user scrolls, indicating their level of interest in the content.
Searches Performed: Any searches the visitor conducts on the website
User-Level Data:
This data requires user identification and can be collected through various methods:
Login Credentials: When users log in to their accounts, information like name, email address, and purchase history becomes available.
Cookies: These small data files stored on the user's device can track browsing activity within a certain timeframe. They can also be used to personalize content based on past interactions. (Note: Cookie usage is becoming more restricted due to privacy regulations).
Email Capture with Opt-In: Encouraging visitors to subscribe to your email list with clear value propositions allows collecting their email addresses and potentially identifying them in the future.
Referral Data: This data identifies where the user came from before visiting your website (search engine, social media, another website).
The conversion curve, with its distinct visitor segments, reveals patterns in user behavior. Analyzing data points like demographics, device usage, and browsing habits allows brands to map visitors onto the curve. Those converting quickly (Bottom 16%) often share characteristics, while those requiring more effort (Top 16%) exhibit distinct patterns. For example:

From the above table, it is evident that visitors from a particular segment of the curve have a lot of similarities. Based on these similarities and other data points more commonalities can be drawn between the visitors depending on the segment they are a part of, such as:
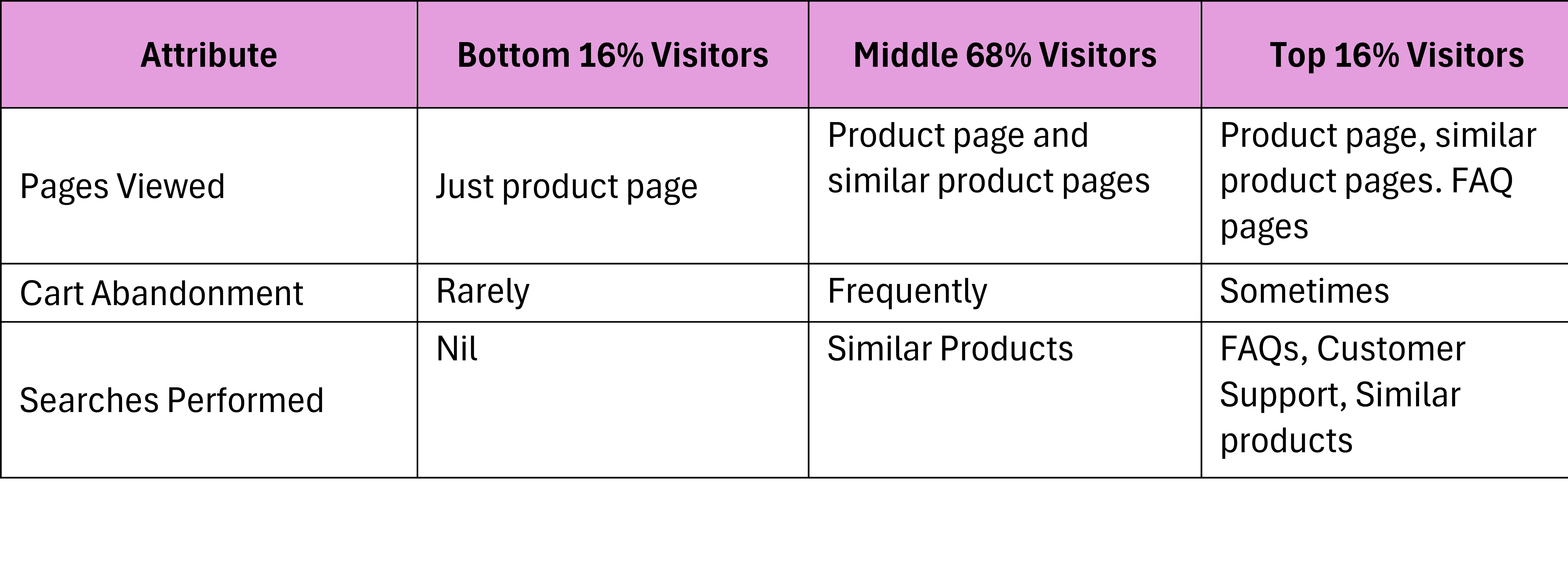
All of this data paints a clear picture: visitors who land on specific segments of the curve demonstrate similar behaviors and likely share common motivations or roadblocks to conversion. It is however important to remember that the visitor conversion curve often won’t present itself in such neat segments as 16%, 68%, and 16%. Visitor behavior typically falls on a spectrum and their characteristics might not always clearly fall into one segment. Price sensitivity might not be a binary factor. Some visitors in the "middle" segment might be price-conscious to a certain degree, while others might prioritize other factors. To deal with this:
Split the visitor base into more segments based on relevant criteria. This could involve segmenting by demographics (age, location), browsing behavior (time spent, pages viewed), or past purchase history (frequency, value).
Even within broader segments, use clustering techniques to identify sub-groups with similar characteristics. This allows brands to tailor their approach even further within each segment.
Instead of looking for definitive lines between segments, focus on identifying trends and probabilities. This could involve analyzing how specific data points influence conversion likelihood. For example, there might be a higher conversion rate for visitors who spend more than 5 minutes on product pages, regardless of segment.
Data Preparation and Fingerprinting
The foundation of personalization lies in data collection, but it's just the first step. For accurate analysis, data needs cleaning. This involves standardizing formats (dates, currencies) and eliminating outliers, like a visitor browsing at 3 AM from a location they aren't actually in (indicating a VPN). Additionally, normalizing data ensures all factors (age, browsing time) are on a similar scale for proper analysis.
To make massive datasets more manageable, techniques like dimensionality reduction can be used to reduce data points while preserving key information. Feature engineering is another technique that creates new data points, like an "engagement score" based on browsing habits.
Another important step is creating a unique identifier for anonymous visitors. This lets you recognize them even if they haven't logged in. Techniques like browser fingerprinting consider factors like the browser version and plugins used to create a kind of digital fingerprint for the visitor's device.
Identifying an anonymous visitor across multiple sessions
Now, when an anonymous visitor returns to the brand’s website and the brand wants to recognize them, even if they haven't logged in, it can be done using probability calculation. Deduplication techniques such as hashing and fuzzy matching are used to stitch together data from different sessions, potentially identifying the same user. Combine the hashed and fuzzed data to create a unique identifier for each visitor. This is called ID stitching.
Mapping anonymous user data also allows for session stitching, connecting multiple sessions from the same anonymous user to build a more complete picture of their journey. This can help identify pain points, optimize the conversion funnel, and create a seamless, personalized experience across multiple visits, ultimately, fostering customer satisfaction and driving growth.
Example
Imagine you run an e-commerce website selling sporting goods. Here's a scenario showcasing fingerprinting and ID stitching with percentages:
Scenario: Sarah (32, uses Chrome on Windows 10) arrives at the brand’s website looking for running shoes. This is the first time she has visited your website, and she is anonymous for the brand.
Session 1: Sarah browses running shoes but doesn't make a purchase. However, she creates a search query for "best running shoes"
Session 2 (2 days later): Sarah returns to your website. She browses running shoes again but still doesn't purchase anything.
Here's how ID stitching helps recognize Sarah across sessions:
5% - Device Only: Only knowing that the visitor uses Windows 10 offers a low chance of identifying them. There are many other Windows 10 users.
15% - Device + Browser: Adding Chrome usage increases the probability, but it's still not very strong. Many people use Chrome on Windows 10.
60% - Device + Browser + IP: Factoring in IP address location narrows down the possibilities. However, it's not foolproof as multiple users can share the same IP range.
85% - Device + Browser + IP + Browser History: By analyzing the visitor's browsing history, which might include pages visited, search queries, or interactions with specific product categories (in this case, running shoes), the probability increases to 85% that the visitor is Sarah.
~100% - Device + Browser + IP + Browser History + Searches Performed: Adding in the searches performed by the visitor in this session along with all the other data points further almost certainly maps the visitor to Sarah.
At this point, with almost certainty, it can be considered a fair assessment that the current visitor is indeed Sarah.
By utilizing these techniques, you can bridge the gap between anonymous and known visitors, enabling personalized user experience by tailoring website content, product recommendations, and marketing messages to individual user needs and improving conversion rates by recognizing returning visitors who previously showed interest, increasing the chance of conversion.
Appendix
Handling Outliers in Visitor Data:
It is essential to remember that data collection is where the process of personalization starts and is not the end. Standardize all dates, currencies and other data formats. Identify and remove any potential outliers. For example:
An IP address from a geographically impossible location. A visitor's IP address indicates they are in New York City, USA, but they are consistently browsing the website at 3:00 AM IST (Indian Standard Time). This time difference suggests the user might be using a VPN (Virtual Private Network) that masks their true location.
Excessively high or low session durations. If the average session duration on your website is 3 minutes, but one visitor has sessions that consistently last for over an hour. This could indicate a bot or script interacting with your website.
Outliers may arise due to various reasons, such as browser extensions or plugins that modify the attributes. To maintain data integrity, eliminate any inconsistencies, errors or irrelevant information.
Normalizing Data:
The data being collected is of different types, such as strings (user agent), numbers (screen resolution), and Boolean values (browser plugin status). To ensure compatibility and consistency for analysis, these different data types may need to be transformed or normalized. Scale the features to a standard range. Categorical data (e.g., browser types) can be encoded using techniques like one-hot encoding or label encoding
Use techniques like min-max normalization (from 0 to 1 or –1 to 1) to ensure all features contribute equally during analysis. Suppose you have user data that includes numerical features like age, income, and time spent on the website (in seconds). These features have different scales and units, which can cause some features to dominate others during analysis or modelling. Min-max normalization can rescale these features to a common range, allowing them to contribute equally.
Use Z-Score normalization to deal with normally distributed data by transforming features with a mean of 0 and a standard deviation of 1. For user data that includes features like session duration and scroll depth, which may follow a normal distribution, Z-score normalization can help standardize these features, making them more suitable for analyses or models that assume normality.
Feature Engineering Data Points:
Since the process of data collection is continuous and large datasets are computationally expensive to analyse; techniques like dimensionality reduction using principal component analysis can help reduce the number of features while preserving most information.
Use feature engineering to enhance analysis. For example, computing an engagement score based on factors like the number of pages visited, time spent on the website, and scroll depth.
Fingerprinting Visitor Data:
When collecting and preparing user data, it is also important to create a unique identifier for a user. This is called fingerprinting.
Analyse browser characteristics like version, plugins, fonts, etc., to create a browser fingerprint.
Layer that up with device fingerprinting, which considers factors like operating system, screen resolution and hardware details.
Follow this up with network fingerprinting which analyses network information like IP address and user agent string.
While not foolproof (since users can change devices or networks), fingerprinting helps identify anonymous visitors across different sessions.
Hashing and Fuzzy Matching:
Hashing (generating unique fingerprints) and fuzzy matching (comparing browsing patterns) help stitch together data points from different sessions. Fuzzing is useful for handling typos or formatting variations, especially in a user-generated field, while hashing is a good technique for efficient duplicate detection. This helps maintain the uniqueness of the user IDs generated by fingerprinting and stitching all data collected.
Keep Reading
Load More